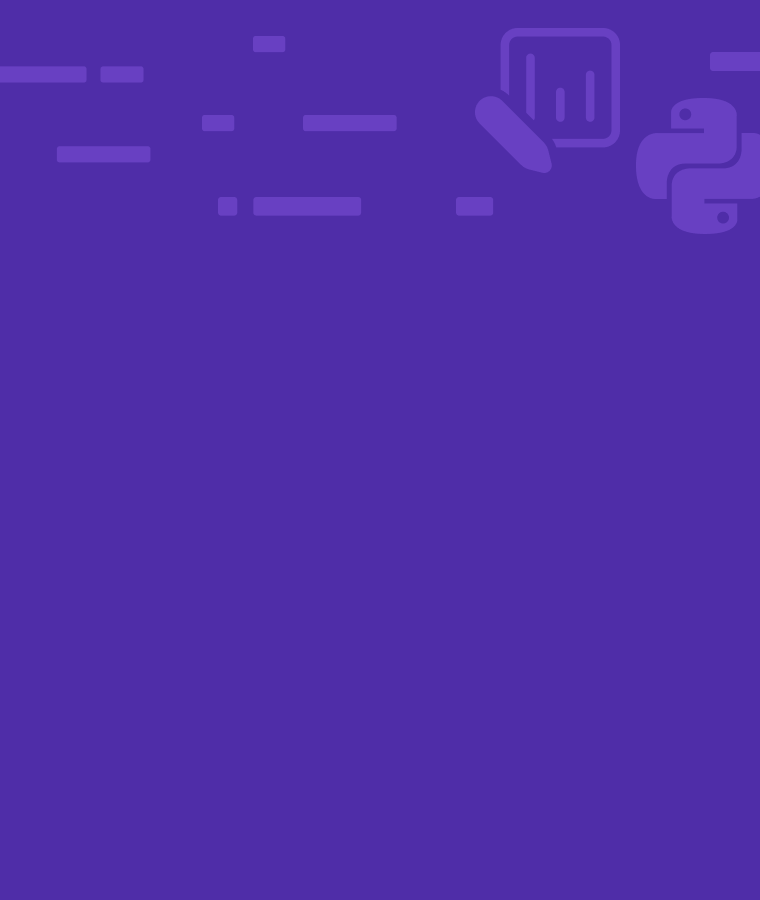
Why Python Is The Most Popular Programming Language For Data Analysis
Discover what Python is and why it has become the most popular programming language for data analysis
If you want to break into data science, sooner or later, you’ll have to learn to code. Writing code is a central part of the daily life of a data scientist.
Data scientists use mathematical and statistical techniques to collect, analyze and extract insights from vast amounts of data.
It would take days, even years, to process all this data if we had to do it manually. Luckily, today we have powerful computers that can do this magic in a matter of seconds. To tell the machines how to perform certain tasks, such as data analysis, data scientists use programming languages.
You won’t go far in your data science career without the proper coding skills. However, figuring out how to start learning to code is one of the most daunting questions for aspiring data scientists.
There are many programming languages that are used in data science. Which one should you learn first?
We recommend starting with Python. Why? Because it’s versatile, ease to use, and perfect for efficiently coding automated tasks and functions.
In this article, we’ll explain:
- what Python is
- why Python is the best option for newcomers to get started in data science
- the most popular Python libraries for data analysis
- the best way to learn Python for data analysis.
What is Python?
Since its launch in 1991, Python has become one of the most popular programming languages in the world. It ranks first in several programming languages popularity indices, such as the TIOBE Index and the PYPL Index. Python is an open-source, general-purpose programming language used in many software development domains, including web development, gaming, blockchain, and, of course, data analysis.

Source: TIOBE
Python was created to be easy to learn, write and understand, and at the same time powerful enough to build any kind of application. Python is often cited as one of the best languages for newer programmers to learn.
This is thanks to its simple syntax (often considered the closest to plain English), gentle learning curve, and structural principles that make it logical and easy to master.
Like other popular programming languages, like Java or C, Python has a large collection of specialized libraries (see below) and frameworks. It’s supported by a huge community of users, who ensure the smooth and solid development of the language.
Why Python for Data Analysis?
When Guido Van Rossum created Python at the beginning of the 90s, he didn’t start out trying to build the best programming language for data analysis. However, thanks to various historical and cultural reasons, in the last 20 years, Python has developed a large and vibrant scientific computing and data analysis community.
There are many reasons why Python is becoming a more popular choice for data analysis tasks compared to other open sources and commercial programming languages and tools, such as R, SAS, or MATLAB.
What makes Python a well-suited candidate for data analysis?
- It’s easy to use. Python’s focus on readability and interpretability makes it the perfect tool for all kinds of data analysis tasks, from collecting and cleaning data, to exploring and extracting insights from huge datasets. All this can be done often in just a few lines of code.
For example, to read a simple .csv file with sales data and transform it into a dataframe (this is usually the first step to conduct a data analysis) you would use the function pd.read_csv(), from the popular Python’s library Pandas:
# read a csv file with pandas import pandas as pd df = pd.read_csv("Desktop/sales_data_sample.csv", delimiter=';', usecols=['ORDERNUMBER','SALES','ORDERDATE','ADDRESSLINE1'], index_col='ORDERNUMBER') print(df) SALES ORDERDATE ADDRESSLINE1 ORDERNUMBER 10107 2871.00 2/24/2003 0:00 897 Long Airport Avenue 10121 2765.90 5/7/03 0:00 59 rue de l'Abbaye 10134 3884.34 7/1/03 0:00 27 rue du Colonel Pierre Avia 10145 3746.70 8/25/2003 0:00 78934 Hillside Dr. 10159 5205.27 10/10/03 0:00 7734 Strong St. ... ... ... ... 10350 2244.40 12/2/04 0:00 C/ Moralzarzal, 86 10373 3978.51 1/31/2005 0:00 Torikatu 38 10386 5417.57 3/1/05 0:00 C/ Moralzarzal, 86 10397 2116.16 3/28/2005 0:00 1 rue Alsace-Lorraine 10414 3079.44 5/6/05 0:00 8616 Spinnaker Dr. [2823 rows x 3 columns]
- Its open-source libraries: The power of Python for data analysis lies in the wide collection of open source libraries and modules that offer entirely new possibilities for fields like data science, artificial intelligence and machine learning. In the following section, we will cover some of the most popular libraries.
- It’s the language for Machine Learning. Machine learning (ML) is a subset of Artificial Intelligence which allows a machine to automatically learn from data without programming explicitly and make predictions. Much of the progress in AI is thanks to Python. Its ability to capture complex ideas in a few lines, together with the large number of existing frameworks, such as Keras, PyTorch or TensorFlow, also make it ideal for this purpose..
Essential Python Libraries for Data Analysis
Any data science tasks you can think of can be done with Python, including:
- data cleaning
- data visualization,
- statistical analysis
- machine learning model deployment,
This is thanks to its wide, community-back ecosystems of libraries and modules. These are some of the most popular ones:
- NumPy. Short for Numerical Python, it’s a popular package for numerical computing in Python. It includes important data structures, such as the NumPy arrays, a large catalog of advanced mathematical functions and associated modules for developing scientific applications.
- Pandas. Since its launch in 2010, pandas has become a central library in Python for data analysis. It provides high-level data structures and functions designed to make working with structured or tabular data intuitive and flexible.
- Matplotlib. It is the standard Python library for creating plots and two-dimensional data visualization.
- scikit-learn. Since the project’s inception in 2007, scikit-learn has become the main general-purpose machine learning toolkit for Python developers. It is built on top of NumPy and SciPy.
- Keras. It is an open-source library designed to train neural networks with high performance.
How to Learn Python for Data Analysis
While many programmers can build web applications with Python, using it for data analysis not only let you to build better applications, but it makes you more appealing to companies looking for versatile Python developers.
Are you ready to begin your data journey? Check out the Python for Data Science course at Sololearn and get started today!
Once you’ve learned the basics of using Python for data analysis, design and create a few test projects of your own!