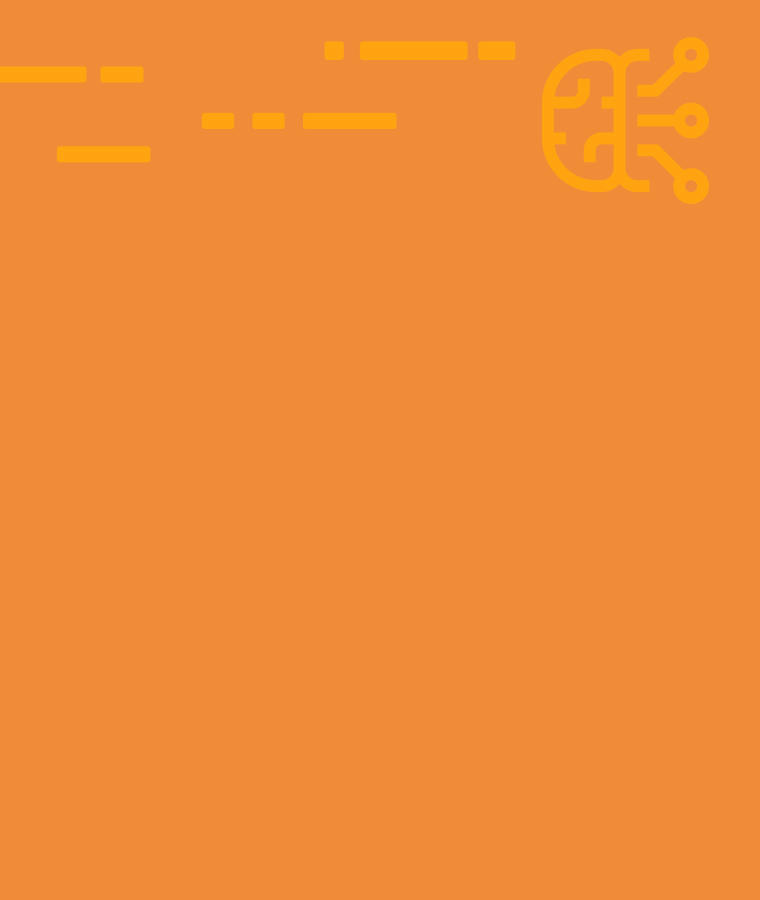
What is Deep Learning?
A lightweight introduction to Deep Learning, one of the most powerful techniques of machine learning
Artificial intelligence (AI) is changing our societies. Computers are creating realities that seemed only possible in science fiction.
For a long time, machine learning was behind most of the developments of the digital revolution. But things started to change in the late 2000s. At that time, a technique called deep learning started to deliver unparalleled results.
Deep learning represents the next step in artificial intelligence evolution. It is a type of machine learning that can use vast amounts of data to make intelligent decisions. As AI expert Andrew Ng said, “[Deep learning] is our best shot at progress towards real artificial intelligence.”
How does deep learning work? How is it being used right now to improve our lives? And how can you learn more about deep learning? We’ll answer these questions in this blog post. Keep reading!
What is Deep Learning?
Deep learning is a subfield of machine learning that employs a type of algorithm called artificial neural networks to learn from large amounts of data and make predictions with high accuracy.
How does deep learning relate to AI and machine learning? Let’s have a look at the following diagram:

AI is a discipline that studies how machines can emulate human intelligence. One of the fields of AI is machine learning. It refers to a set of techniques to teach computers how to learn from data and perform tasks with minimal human intervention.
Finally, deep learning is a subfield of machine learning that focuses on the use of artificial neural networks.
How Deep Learning Works?
To understand what makes deep learning so powerful, let’s study how it works. This will allow you to understand what makes it different from other types of machine learning algorithms.
Deep Learning Uses Multiple Neural Networks for Faster Learning
An artificial neural network is a system that aims to mimic human brains. It works by simulating a large number of processing units that resemble a network of neurons. The processing units are sorted into different levels, called layers. A simple neural network consists of one input layer, one hidden layer, and one output layer.
When the model has more than one hidden layer, we called it a “deep” neural network. The more hidden layers, the deeper and more complex the neural network will be.
In artificial neural networks, statistical information propagates from one neuron to every neuron in the next layer. Each neuron has a weight, which controls the way information flows. Each time the model makes an incorrect prediction, the weights are recalculated. After several iterations, the accuracy of the model will improve.
Deep Learning Uses More Data and Computing Power to Learn
Another feature of deep learning is its use of vast amounts of data and computing power.
The majority of machine learning algorithms can run on a regular laptop. That is not the case for neural networks. They usually need hundreds of terabytes of data to learn and perform a task — much more than a single computer typically stores.
Equally, given the complexity of the system, running a neural network also requires a lot of computing power. In recent years, graphics processing units (GPUs) have been used to improve the efficiency of deep learning systems. Regular computers rarely come with powerful GPUs and getting them is expensive.
This is why deep learning systems are usually built in large data centers that have access to large amounts of data storage and computing power.
Why is Deep Learning so Powerful?
Deep learning is behind some of the most important milestones in artificial intelligence. Here are the some of reasons why:
- Neural networks can handle unstructured data. Machine learning models are suitable for structured data stored in tables. However, they are not designed to deal with unstructured data, such as:
- Images
- Audio files
- Video files
By contrast, neural networks are capable of dealing with both structured and unstructured data.
- Neural networks can handle large datasets. Thanks to the adoption of GPUs in deep learning, neural networks can process large amounts of data with incredible speed.
- Neural networks have unbeatable accuracy. Neural networks are complex and powerful algorithms. They are capable of performing all kinds of tasks, with incredibly accurate results.
Applications of Deep Learning
Neural networks are the go-to algorithms to process unstructured data. The rapid development of deep learning has resulted in a large catalogue of new applications, including:
- Computer Vision. Computer vision is an interdisciplinary field that focused on how computers can gain high-level understanding from digital images or videos. Deep learning is at the forefront of the discipline. Some of the applications powered by neural networks are:
- Recognition of objects in images
- Reparation or manipulation of damaged images
- Image search based on keywords.
- Speech Recognition. Deep learning is a core element in modern smartphones. One example is popular virtual assistants, such as Alexa and Siri. These assistants rely on neural networks that continually improve themselves by recognising and collecting voices from the millions of people who use them every day.
- Autonomous cars. Deep learning is the force that is bringing autonomous driving to life. A complex system of neural networks is used to ensure secure driving. The model uses tonnes of data collected in real-time from the environment. Data from cameras, sensors and geographic maps are helping to create sophisticated models so that machines are able to navigate through traffic.
Learning More About Deep Learning
At first, deep learning can seem like an intimidating subject to learn. It’s true that it is a very broad and complex field, and that some very intelligent people are working on it every day. But this doesn’t mean that you can’t get started learning about it and even working toward a career in it.
As mentioned, deep learning is a specific application of machine learning. In SoloLearn’s free Machine Learning course, you’ll use Python to explore the basics of machine learning, including classification, decision trees, and even neural networks. These concepts will put you well on your way to understanding deep learning.
Because deep learning relies on data, you may also want to explore the Data Science with Python course. These data analytics concepts are critical to understanding how data is used in machine learning and AI.